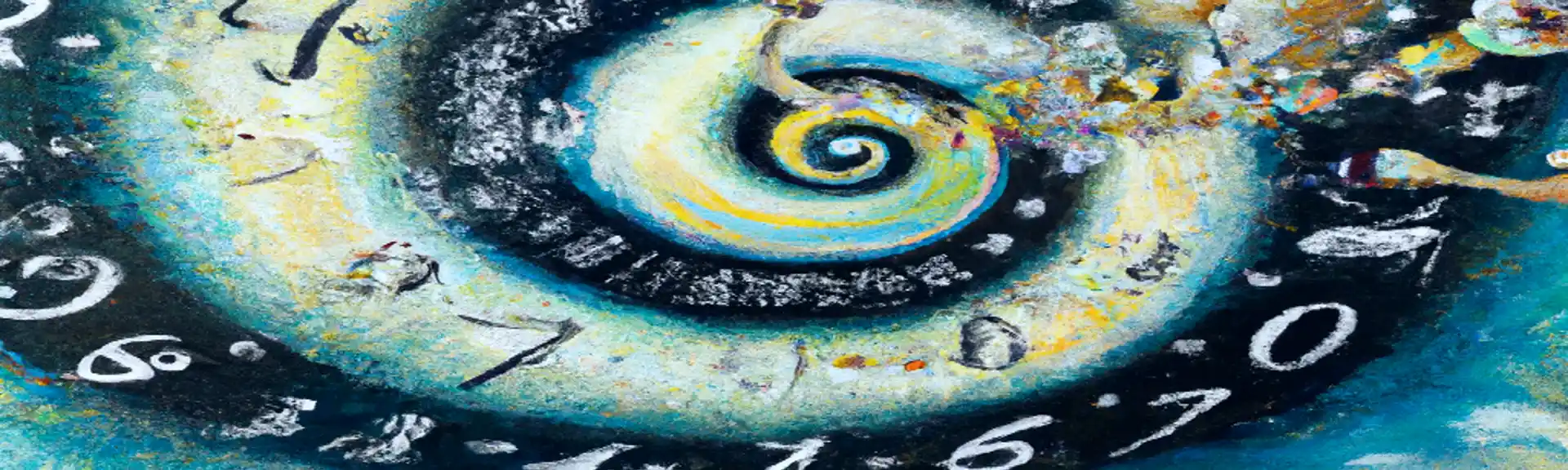
Myths about learning and teaching maths continue to plague classroom practice despite consistent lack of evidence demonstrating positive outcomes for students.
In this paper, leading education researchers Sarah Powell, Elizabeth Hughes and Corey Peltier debunk seven commonly-held myths about teaching maths – (1) conceptual then procedural understanding, (2) teaching algorithms is harmful, (3) inquiry learning is the best approach, (4) productive struggle is important, (5) growth mindset increases achievement, (6) executive function training is important, and (7) timed assessments cause mathematics anxiety.
The authors explain how mathematics education can become more evidence-based, before providing a series of recommendations for improving maths teaching practices to best support student learning. As they conclude, we are doing a disservice to students when unsubstantiated practices are used in place of ones with substantial supporting evidence and greater likelihood of success. Sharing and following the educational science will mean more students succeed.
Myths That Undermine Maths Teaching
Competency with mathematics is essential for success in school and career.[1] [2] That is, students who take and succeed in higher-level mathematics coursework are more likely to get into college,[3] [4] graduate from college,[5] and earn more income as adults.[6] The pathway for success with mathematics begins quite early in a student’s life, most often before a student starts formal schooling. Even before the age of 5, students perform differently on mathematics tasks,[7] [8] and these differences in learning readiness contribute to some students experiencing more challenge learning mathematics as they begin formal schooling.[9] [10]
Mathematics builds in complexity; perhaps that is why early performance in mathematics relates to future performance. For example, mathematics at age 5 predicts later mathematics performance through age 15[11] as mathematics at age 8 relates to mathematics performance at age 12.[12] Furthermore, mathematics around ages 10-12 predicts mathematics 5 years later.[13] These patterns show that when students succeed with mathematics at an earlier grade level, they continue to perform well in subsequent grade levels. But the opposite is also true – when students struggle with mathematics at an earlier grade level, they may continue to struggle with mathematics later on. For students with disabilities, mathematics performance is, on average, lower than students without disabilities.[14] The difference in mathematics performance for students with and without disabilities widens from grade level to grade level,[15] creating wider and wider disparities as students age.[16] Fortunately, research shows that access to high-quality instruction and intervention can change trajectories of student outcomes across a school year.[17] [18] Therefore, high-quality mathematics instruction in schools is essential to alleviate the difficulties that many students face with mathematics.
The term ‘high-quality mathematics instruction’ could be interpreted in dozens of ways. In this paper, we propose that high-quality mathematics instruction should be instruction that is driven by evidence from clinical studies or school-based studies.[19] Evidence is data, most often from students, teachers, or schools, that shows a practice contributes to increased mathematics knowledge.[20] High-quality instruction should be based on current and comprehensive evidence rather than antiquated or unsubstantiated myths. Unfortunately, there are quite a few prevailing myths that are pervasive in conversations about the teaching of mathematics. We are doing a disservice to our students when these unsubstantiated practices are used in place of ones with substantial supporting evidence and greater likelihood of success. In this paper, we describe several prevalent myths and provide support to debunk them, in an effort to change conversations and maths teaching practices to best support student learning.
Maths Teaching Myth: Conceptual Then Procedural Understanding
Myth
One commonly-held myth amongst educators is that students should not be exposed to procedural instruction until they have demonstrated adequate conceptual understanding of specific mathematics content. Conceptual knowledge is an understanding of concepts (e.g., magnitude of numbers, regrouping), whereas procedural knowledge is often described as the steps necessary to solve a problem.[21] This myth may come from pushback to procedural learning,[22] from resources that state conceptual knowledge should be developed prior to procedural knowledge,[23] or from descriptions of a concrete-representational-abstract learning sequence in which the abstract learning is presented last.[24] As noted by Rittle-Johnson et al,[25] no empirical evidence supports claims about an instructional sequence (e.g., conceptual before procedural) that leads to improved student outcomes.
Truth
The truth is that conceptual knowledge and procedural knowledge work in tandem and are often intertwined.[26] Conceptual knowledge supports procedural knowledge and procedural knowledge supports conceptual knowledge. As an example, in 1999 Rittle-Johnson and Alibali[27] worked with elementary students on solving different types of equations. When provided with conceptual instruction, students demonstrated improved knowledge on measures of conceptual and procedural knowledge. When provided with procedural instruction, students showed improvement on conceptual and procedural measures. Although the authors determined a slight asymmetrical relationship between the influence of conceptual and procedural instruction, in 2015 Rittle-Johnson et al.[28] suggested both conceptual and procedural knowledge are necessary and “there is not an optimal ordering of instruction” (p. 595).
As described by Schneider et al.,[29] there is a bidirectional relationship between conceptual and procedural knowledge. In a study focused on equation solving, Schneider and colleagues learned that conceptual knowledge and procedural knowledge both contribute to success with equation solving. These two types of knowledge overlap – that is, they are not entirely separate constructs. The authors also learned that conceptual knowledge predicted later conceptual and procedural knowledge. In turn, earlier procedural knowledge predicted later conceptual and procedural knowledge.
In Maths Teaching
To ensure development of conceptual and procedural knowledge in tandem, consider the following:
(1) Model and practise conceptual understanding as part of solving mathematics problems. For example, when teaching students to solve mathematics word problems, emphasise the structure or schema of the different word problems.[30] These structures represent the conceptual underpinnings of the word problems (e.g., putting amounts together versus comparing amounts). Hands-on tools, drawings, and other representations can help students understand the concepts and distinguish among them.
(2) Model and practise procedural understanding as part of solving mathematics problems. For example, provide step-by-step demonstrations of how to solve an addition, subtraction, multiplication, or division problem, how to find common denominators, or how to solve an algebraic equation.[31] Procedures are often equated with algorithms, but procedural knowledge includes more than understanding and applying an algorithm.[32]
(3) Explicitly connect conceptual and procedural knowledge. Teaching these together can help strengthen the other over time.[33] When connecting conceptual and procedural knowledge, monitor student learning and be aware of student limits on cognitive load and working memory. If using the concrete-representational-abstract in mathematics instruction, ensure it is interpreted as a framework (in which several components may be in use at any one time) instead of a sequence. As an example, if teaching about the Pythagorean theorem, an educator could use hands-on tools to have students create right triangles. The class could discuss the sides (a, b, c) and angles of the triangles and the relationships among a, b, and c. An educator would also introduce a formula (a2 + b2 = c2) and engage in modeling and practice about how to use the formula to solve for unknown quantities in problems. The conceptual knowledge helps students understand the properties of triangles and when to use the Pythagorean theorem, and the procedural knowledge assists with the solving of problems – both are necessary for success with such problems.
Maths Teaching Myth: Teaching Algorithms is Harmful
Myth
Another myth is that students should not be taught algorithms. An algorithm is a step-by-step procedure for solving a problem; often the term algorithm is applied to solving addition, subtraction, multiplication, and division problems beyond the mathematics facts. Algorithms would pertain to problems such as 145 + 98, 2,034﹣1,670, 78 × 42, or 1,200 ÷ 15. This myth may have developed based on a study in which authors suggested to “stop teaching the algorithms, and instead, encourage children to make the mental relationships necessary to build number sense”.[34] In a rebuttal to this study, Fischer et al.[35] noted that Kamii and Dominick[36] did not allow students who learned a standard algorithm to use it and only compared their performance to students who created their own algorithm. Therefore, they suggested the Kamii and Dominick research could not support the claim to stop teaching algorithms. Additionally, over the last two decades, students have been encouraged to develop their own reasoning and strategies and develop flexibility with mathematics,[37] therefore, some educators may have moved away from teaching algorithms.
Truth
Mathematics standards have emphasised that students should learn algorithms alongside the conceptual meaning of each of the algorithms.[38] Furthermore, meta-analyses have noted that teaching students explicitly how to solve mathematics problems leads to improved mathematics outcomes over encouraging students to create their own mathematics knowledge.[39] In a research study, Torbeyns and Verschaffel[40] demonstrated that students, after a year of practising an algorithm, could apply the algorithm correctly and preferred using the algorithm. When students did not use an algorithm, they often employed inefficient strategies to solve computation problems. In a comparison of standard algorithms to alternate algorithms, Norton[41] worked with students across ages 9 to 12 on a measure of addition, subtraction, multiplication, and division computation. He determined that, across operations, students who employed standard algorithms were more likely to solve each problem correctly than students who used alternate algorithms.
In Maths Teaching
When teaching students algorithms, consider the following:
(1) Teach an algorithm in tandem with a focus on conceptual knowledge. As suggested by Fischer et al.,[42] algorithms are helpful for successful mathematics students but algorithms must be taught alongside conceptual knowledge so students know when and how to apply each algorithm. For example, if modeling how to use an algorithm to add 178 + 346, emphasise place value concepts of hundreds, tens, and ones and emphasise the concept of regrouping (i.e., 13 is 1 ten and 3 ones). As described earlier in this paper, conceptual and procedural knowledge should develop concurrently.
(2) Check student background knowledge. To use an algorithm well, students have to have a strong understanding of numbers and place value.[43] That is, ensure students have a foundation in understanding what it means to add, subtract, multiply, or divide before introducing an algorithm.
(3) Model and practise. In a study with university students, many had difficulty with the algorithms after years of not practising them.[44] Students often apply the wrong operation within computation;[45] therefore, practise identifying the operator symbol and determining which algorithm to apply may be particularly important. Many algorithms may need to be explicitly modeled and practised, especially for students who experience mathematics difficulty,[46] [47] so it is important to determine the timing of such modeling. For students with mathematics difficulty, more practice opportunities (than students without such difficulty) may be necessary to become efficient with an algorithm, so carve out practice time for students.[48]
(4) Consider factors important for computation with algorithms. Researchers have identified mathematics fact skill (i.e., 4 + 5, 13﹣9, 8 × 7, or 45 ÷ 9), working memory, and processing speed as predictors of computation performance.[49] [50] Providing more practice of mathematics facts may lead to improved computation performance. While working memory practice may be important for some aspects of mathematics,[51] [52] research is on-going about best practice for engaging working memory within mathematics. Therefore, being aware of the working memory and processing speed limitations of students could be a helpful starting point for design of instruction related to the algorithms.
Maths Teaching Myth: Inquiry-Based Learning is the Best Approach to Introduce and Teach Mathematics
Myth
The myth remains that inquiry-based learning should be the primary method used to teach mathematics.[53] The first issue with this myth is the assumption that inquiry-based instruction should be the standard method of learning. Data from international assessments (e.g., PISA) suggests that many students experience challenges learning mathematics. In addition, educators are going to see the impact of recent and unprecedented interruptions to instruction on students’ mathematics performance for years to come. A majority of students will benefit from more structured initial instruction. Next, inquiry-based learning is suggested to increase achievement, curiosity, and interest in mathematics, as well as promote motivation to solve new and novel problems, yet we do not have sufficient data to support these claims.
Truth
Students have difficulty with learning when instruction is misaligned with student learning needs and readiness.[54] While some students may thrive with true inquiry-based learning, their success is an exception rather than the standard outcome. In fact, decades of research evaluating effects of inquiry-based learning and guidance demonstrated that more specific supports and guidance have been more effective than inquiry without supports in a wide range of contexts.[55] [56] [57] [58] [59] De Jong and Van Joolingen[60] reported that the forms of inquiry that were most beneficial were those that also included access to relevant information, in addition to support to structure inquiry and monitor progress — all elements that align with explicit instruction.[61]
Essentially inquiry-based learning is reactive rather than proactive to student readiness. Lazonder and Harmsen[62] noted that many of the supporting scaffolds reported in studies in their meta-analysis were added ‘ad hoc’. Rather than assuming inquiry-based learning is the best approach to introduce and teach mathematics, it is more appropriate to design instruction based on content, existing evidence of effectiveness, and likelihood of success considering student strengths and learning readiness. To do this, consider student readiness to engage in a learning activity. Novice learners, for whom the concepts and procedures are new or not-yet understood benefit from explicit instruction more than inquiry-based learning.[63] With explicit instruction, the educators consider the scope and sequence of mathematics, building and connecting new concepts with previously learned concepts, and guide the learning process by modeling the skill, providing scaffolded practice, and finally encouraging independent practice and application of skills.[64] Metaphorically, a builder wants to build a birdhouse. They need the appropriate tools and materials to complete the project. If the builder has the materials, but not the tools, the builder will likely not be successful in the process or may build something that takes more effort and is less effective.
In Maths Teaching
To provide all students with high-quality mathematics instruction, consider:
(1) Teach with evidence-based practices first. One evidence-based practice with a large corpus of evidence includes explicit instruction.[65] [66] [67] [68] [69] Explicit instruction is a combination of modeling, practice, and feedback.[70] [71] Explicitly teaching skills students are initially acquiring affords students to be successful applying skills later to new and novel concepts provided through inquiry.
(2) Rather than offering true inquiry, provide modified inquiry learning with built-in scaffolds and support for student success. Explicit instruction is important when the discovery process may be inaccurate, incomplete, inefficient, or inadequate,[72] which is often for many students. Anticipating student challenges during inquiry and proactively providing scaffolds and supports will help students make connections. Incorporate evidence-based practices including: guided notes[73] to support learners progression through the task, self-regulated strategy development (SRSD) to support self-regulation in multi-step problem solving tasks,[74] and incorporate teaching how to use concrete manipulatives[75] or the concrete-representational-abstract instructional framework[76] to develop conceptual understanding.
Maths Teaching Myth: Productive Struggle is Important
Myth
Productive struggle is when educators present students with hard and complex mathematics problems that require students to grapple with problem solving and persevere through missed steps or failures to determine a solution to the problem. It is believed that this struggle develops student “grit,” fosters deeper understanding, and promotes creative problem solving. Productive struggle is anchored in the assumptions that (a) the activity will be challenging and difficult for the student, (b) students will self-regulate through trial and error problem solving, (c) engaging in struggle focuses on concept and problem-solving process,[77] and (d) students are motivated to persist- rather than just solve for the right answer.[78] At face value the assumptions are reasonable, but research draws attention to the different ways productive struggle is problematic.
Truth
The struggle many students experience is frustrating and wasteful rather than productive. As explained by Hiebert and Grouws,[79] “do not use struggle to mean needless frustration or extreme levels of challenge created by nonsensical or overly difficult problems” (p. 387). Much of the difficulty with productive struggle is how educators interpret the level of struggle. Many students find this process excessively frustrating, overwhelming, and a fruitless practice of practising and applying misinformation. Hiebert and Grouws[80] suggested “struggle to mean that students expend effort to make sense of mathematics, to figure something out that is not immediately apparent” (p. 387). In the following paragraphs, we expand upon each of the assumptions behind the myth of productive struggle.
Similar to inquiry-based instruction, the impact on student outcome is not as favourable as the intent of the instructional process. Addressing the underlying assumptions: The practice of providing a ‘hard’ problem to solve suggests that the task is beyond reasonable reach of students. National Council of Teachers of Mathematics[81] shares that students should experience appropriate challenges; but, what is appropriate? Appropriate requires substantial understanding of concepts and procedures in order to generalise them to new and novel situations. Activities that require application of skills students do not have are an ineffective use of instructional time. Students actually need considerable prior knowledge to self-regulate novel problems.[82] Explicit instruction is more appropriate for initial and acquisition instruction while productive struggle may be more appropriate for generalization when students are proficient in requite skills necessary to adequately solve the problem.
Next, self-regulation is effective when students are self-regulating their behaviours and processes, but self-regulation does not replace skill and substantial support is needed to scaffold to correct missteps. Redirecting instructional effort from inquiry-based instruction to self-regulated strategy development (SRSD), an evidence-based practice that has substantial research supporting student outcomes in mathematics and other academic areas, is a more efficient use of time and resources.[83] [84] SRSD focuses on supporting students successfully engaging in higher-level cognitive processes, self-monitoring their own learning process, and developing positive learning attitudes. In this process, students are taught to set goals, monitor learning behaviours, engage in positive self-talk, and reinforce successes. Explicit instruction components embedded in the process contribute to SRSD’s effectiveness (e.g., Hughes & Lee, 2020).[85]
There is no evidence that struggling with a mathematics concept increases the problem-solving process in such a way that impacts achievement outcomes. While resources on how to “support” productive struggle are readily available on the Internet, research to support this concept is weak and unsubstantial at best (e.g., Kapur, 2014),[86] unlike evidence-based practices, such as those mentioned throughout this paper, that have substantial evidence supporting favourable achievement outcomes. Conceptual and procedural knowledge are important and bidirectional.[87] Essentially, they should be taught simultaneously. Drawing mathematical connections is important to build conceptual understanding.[88] Productive struggle does not support students building connections between relevant information and concepts. When productive struggle and explicit instruction were compared in two randomised controlled trials, which are the ‘gold standard’ in research design, students who received explicit instruction prior to problem solving activities outperformed students who engaged with problem solving prior to explicit instruction.[89]
Finally, productive struggle is perceived as motivating because students are not just replicating a procedure. An authentic application to mathematics can be motivating to students, especially if the problem to solve is one that they value as interesting or important. However, there are a few things to consider regarding motivation to learn mathematics. Student motivation to learn is important, but does not replace or make up for lack of skill. As such, students who are motivated to learn and have the necessary skills to be successful are more likely to outperform students who are motivated but have not learned the skills required for the task,[90] suggesting motivation to learn will not make up for students’ under-preparedness due to lack of previous instruction.
In Maths Teaching
Here are several suggestions related to productive struggle:
(1) Consider the scope and sequence as well as learner readiness when presenting problem-solving activities. Students should be set up to be successful with mathematics. Order of activities matter, with effective instruction preceeding challenging application.[91]
(2) Be sure students have a firm understanding and error-free application of requisite skills to solve novel problems.
(3) Have instructional scaffolds in place that can be removed if or when students no longer need them. It is better to have the scaffolds in place and remove them than to try and add them in after students have already struggled and possibly become confused and frustrated. Using worked examples are one way to support procedural skills and conceptual understanding.[92]
(4) If you want to pique students’ interest at the beginning of the lesson by presenting a challenging and novel problem, allow limited time for exploration (e.g., approx. 5 min, no more than 10 min). Disclose to students before the lesson that this is an exploratory activity and the purpose is to explore the concept rather than solve for the ‘right’ answer at this time.
(5) Motivate students by effectively teaching them the skills to be successful.
Maths Teaching Myth: Growth Mindset Increases Achievement
Myth
Growth mindset theory emerged from two theories from motivational research – attribution theory and achievement goal theory. Attribution theory postulates that individuals’ perceptions (or attributions, hence the name) for why they experienced success or failure when engaging in an event will shape their reaction to the effect moving forward.[93] For example, a student may attribute their success to them being smart versus another student may attribute their success to the effort and practice they put into learning the skill. This differentiation in attributes may shape students’ reaction to similar events in the future or how they handle success and failure. Achievement goal theory postulates that the nature of an individual’s goal will impact their attributions to events — thus the tie back to attribution theory.[94] Students who have a task goal, for example an aim to improve learning, will have more positive attributes and respond better to failure than students who have ego focused goals, for example aiming to perform better than others.
These theories build the foundation for mindset theory and the focus on a growth mindset.[95] Mindset theory suggests individuals may have a growth mindset and believe that personal characteristics are malleable and can be changed, or a fixed mindset and the belief these characteristics are unchangeable. The theory posits that individuals with a growth mindset are more willing to set goals and put forth effort to enhance learning and growth in specified areas because of the belief that growth is possible. Conversely, individuals with a fixed mindset will put forth less effort and set lower goals because of the belief these characteristics are fixed. So where is the controversy? The question lies at whether instructional time should be allocated specifically on mindset training and whether this decision is likely to result in meaningful mathematics gains.
Truth
The current research has shown minimal evidence to support stand-alone growth mindset interventions. An initial efficacy study demonstrated minimal gains on grade point average and mathematics course grades;[96] however, a replication attempt failed to show promising results.[97] A recent randomised control trial assigned third graders who demonstrated need for mathematics intervention to three separate conditions: (a) business as usual, (b) fraction intervention, and (c) fraction intervention plus growth mindset training. Results revealed no difference in mathematics learning gains between the stand alone fraction intervention in comparison to the fraction intervention plus growth mindset.[98] So where does this leave educators based on growth mindset theory?
In Maths Teaching
Several suggestions include the following:
(1) Focus mathematics instruction on building mathematics skills.
(2) Acknowledge that student engagement during instruction and willingness to put forth effort to engage in mathematics tasks is needed. Thus, embedding high-quality instructional tactics focused on reinforcing student engagement, task persistence, and self-regulation while also delivering high-quality mathematics instruction is critical. Three low-intensity and easy to implement practices focused on this endeavour include (a) behaviour specific praise statements, (bteaching students a self-monitoring procedure for academic skill performance or behaviour performance (e.g., on-task), and (c) goal setting.
Maths Teaching Myth: Executive Function Training is Important
Myth
Executive functions is theorised as a set of cognitive skills that allow people to direct behaviour toward the attainment of a goal.[99] [100] What are the core cognitive skills that comprise executive functions? Short answer, it is messy and research teams have taken different theoretical approaches to operationally defining this construct! Three skills typically included in definitions are inhibition, updating, and switching. Behavioural inhibition is the ability to inhibit an initial response in favour of a subdominant response. For example, a student working through addition and subtraction mixed skills may see ‘4’ and ‘3’ and immediately want to say ‘7’ but inhibiting this response to instead inspect the operation sign “-“ will allow the child to provide the correct response. Updating is the task of evaluating new information being presented and determining its relevance to achieving the goal at hand. An excellent example of this can be found in math word problems (i.e., story problems). The math situation presented in the word problem often contains relevant and irrelevant information that students need to sift through – updating would be hypothesised as being a critical skill to achieve this goal. Last, switching is the ability to shift one’s attention to different information or switch between strategies being employed or switch between different tasks. One salient example of when switching would be relevant is using the standard algorithm within multi-digit multiplication. Students must shift between using multiplication and addition throughout the process.
There are two related myths related to executive functioning and mathematics instruction. First, there is a belief that executive functioning is more influential on students’ mathematics achievement than other academic domains. A meta-analysis evaluated the current empirical data evaluating correlations between executive functioning and reading on mathematics achievement, and the authors found the correlation to be similar across both outcomes.[101] Thus, executive functioning is not more influential on mathematics achievement than reading achievement.
The second belief is that stand-alone interventions targeting executive functioning will transfer to improvements in mathematics outcomes. A meta-analysis evaluated the experimental evidence on the effects of executive functioning interventions on a variety of outcomes.[102] So, what did they find? An intervention targeting a specific aspect of executive functioning (e.g., inhibitory control, working memory) demonstrated improvements in that specific skill, but limited evidence of transfer to a related skill. For example, an intervention targeting working memory may show improvements in working memory (using the same measurement instrument), but the learner would not show gains in other aspects of executive functioning (e.g., cognitive flexibility, behavioural inhibition). Second, the authors found no evidence of far-transfer to academic skills. For example, training working memory would not then lead to increased learning in mathematics facts. To summarise, stand-alone executive functioning interventions have no evidence to yield mathematics learning gains.
Truth
It is likely cognitive factors, such as executive functioning, will be correlated with mathematics achievement.[103] However, the current empirical evidence is messy because many experiments have failed to control for a host of learning histories, environmental factors, and other biological components when evaluating the correlation between executive functioning and mathematics achievement. Jacob and Parkinson[104] identified only five of the 67 studies included in their meta-analysis rigorously controlled for other variables when evaluating the relation between executive functioning and mathematics (or reading) achievement. Only one of the 13 investigated associations between executive functioning and academic achievement remained statistically significant after controlling for other variables likely to impact academic achievement. Thus, the causal link between executive functioning and mathematics achievement is severely limited given the current evidence.
In Maths Teaching
Consider the following related to executive functions within mathematics:
(1) Teach explicitly. Unambiguous, systematic, and explicit instruction reduces the likelihood student differences in executive functioning will impact learning. The core components of explicit instruction aim to (a) segment complex skills, (b) focus student attention on salient concepts, (c) systematically fade supports, (d) high levels of student responsiveness, and (e) provide meaningful practice opportunities.[105] [106]
(2) Segment skills and use clear language. A focus on segmenting complex skills into subskills and explicitly highlighting salient concepts through clear and concise language (and perhaps supported through visuals or manipulatives) will reduce the impact executive functioning needs to play in accessing the learning environment. See Hughes et al.[107] and Powell et al.[108] for examples of clear and concise mathematics language in elementary and secondary mathematics.
(3) Allow for high levels of opportunities to respond, which are connected to positive student outcomes,[109] and provide students with meaningful practice. If students are task engaged and building automaticity in the core skills, this will reduce the working memory load as the composite skills are used for more complex skills later in the year.
Maths Teaching Myth: Timed Assessments Cause Mathematics Anxiety
Myth
As the name implies, timed assessments consist of students answering mathematics items under timed conditions. The myth suggests that having students solve mathematics items under timed administration protocols will cause a subset of students to develop mathematics anxiety. This myth is multifaceted in why it is persistent in mathematics education. First, educators are concerned that having students answer difficult mathematics items under timed conditions will produce stress and anxiety that will ultimately manifest into a negative affect towards mathematics generally and mathematics anxiety. Others use this claim of timed assessments causing mathematics anxiety as part of an attack against being ‘quick’ or ‘speedy’ at mathematics as a goal for mathematics education and instead the onus should be placed on deep conceptual understanding and flexibility in mathematical thinking. However, research consistently shows timed assessment is beneficial from both a measurement standpoint and an instructional standpoint. Teachers are rightfully wary of timed assessment because when used ineffectively it yields useless data and is an ineffective instructional technique.
Truth
Before diving in, let us consider a hypothetical situation. An educator administers a maths multiplication fact sheet to all students in the class. All students get the same exact sheet. Before the educator starts the timer, they say, “It is important to master our maths facts. If you get below [X] correct, then we will spend the first 5 minutes at recess practising.” As students work on the sheet you see students quickly slapping their pencil down and flipping their paper over to signify, they finished. Other students quickly look at them and frantically get back to their sheet. As the timer rings the educator yells, “Times up, trade with your shoulder partner.” The educator has students exchange sheets with a peer. As the educator reads off the answers the peer scores the sheet. The educator then posts everyone’s score on a classroom board to track student progress.
This hypothetical is what many conjure when thinking of timed assessment. There are many issues with correct implementation of timed assessment in this hypothetical: (a) assigning same problems to all students, (b) removing a reinforcing activity based on skill performance, (c) using a problem set with too low a ceiling so students see others finish first, (d) using peer grading to promote normative comparison, and (e) public posting of performance.
The truth is there is no causal evidence that timed assessments will produce mathematics anxiety. When used appropriately, timed administration is a valuable instructional technique. From a measurement standpoint, capturing fluency of mathematics performance is a more sensitive and accurate evaluation of student performance than solely relying on accuracy.[110] A necessary point to reiterate here is that fluency is defined as speed and accuracy,[111] for example, how many correct mathematics items were solved within 1 min. Capturing correct responses under timed conditions is the basis for curriculum-based measurement, which is a foundation for the screening and progress monitoring systems schools use as part of tiered models of instruction.[112] From an instructional standpoint, timed assessment is just retrieval practice opportunities. Students who can effortlessly retrieve declarative facts required for procedure or application tasks will be much more likely to be successful on the target task. This can be tied back to cognitive load theory.[113] If declarative facts are not known, working memory will be depleted by attending to the declarative facts and unable to focus on the details required for the procedure or application task. By incorporating a timed component and using goal setting (i.e., can you beat your own score from last time?) students will aim to retrieve the fact or procedure and apply it repeatedly during the timed trial.
In Maths Teaching
Here are several suggestions related to timed assessment:
(1) For educators providing core instruction (i.e., Tier 1) it will be useful to identify the class median performance on discrete math skills (e.g., sums to 20, double digit addition, etc). The class median performance will guide teachers to identify the skill that is situated in the classwide instructional range. By using classwide data to inform instruction, students will be more likely to encounter math tasks that are known but have a slow response time, thus perfect for fluency practice (to read about the instructional hierarchy, see Burns et al., 2010).[114] From an instructional standpoint, explicit timing involves students practising mathematics items under timed conditions.
(2) Administer timed assessments as low-stakes activities. For example, do not tie the assessment to a grade, consequences of scores should not include removal of desired activities, and avoid peer-to-peer comparisons.
(3) Embed goal setting as part of the process. Have the students graph their score (on a graph that only they can see — no public sharing of graphs). Have the students aim to beat their previous score — because instruction is occurring on these skills this should be likely.
(4) If administering timed assessment class wide, ensure the floor is high enough for all students. This means enough items need to be included that no student should finish all items on the sheet in the time frame. Explicitly telling students this expectation will reduce concerns that all items were not solved.
Conclusion
Myths about learning have been around for a long time and continue to plague classroom practice despite consistent lack of evidence demonstrating positive outcomes for students.[115] In this paper, we addressed seven myths:
(1) conceptual then procedural understanding;
(2) teaching algorithms is harmful;
(3) inquiry learning is the best approach;
(4) productive struggle is important;
(5) growth mindset increases achievement;
(6) executive function training is important; and
(7) timed assessments cause mathematics anxiety. We described each specific to the teaching and learning of mathematics and presented a counter position based on current knowledge in the field of education. Letting go of instructional myths may not be easy, as they are often repeated as truth and accepted at face value.
To provide all students with high-quality mathematics instruction, we provided the following suggestions:
(1) Conceptual and Procedural Understanding:
- Model and practise conceptual understanding as part of solving mathematics problems.
- Model and practise procedural understanding as part of solving mathematics problems.
- Explicitly connect conceptual and procedural knowledge.
(2) Teach Algorithms:
- Teach an algorithm in tandem with a focus on conceptual knowledge.
- Check student background knowledge, such as understanding of numbers and place value.
- Model and practise algorithms
- Consider factors important for computation with algorithms, such as mathematics fact skill, working memory, or processing speed.
(3) Teach Using Evidence-Based Practices:
- Teach with evidence-based practices first.
- Rather than offering true inquiry, provide modified inquiry learning with built-in scaffolds and support for student success.
(4) Understand Limitations of Productive Struggle:
- Consider the scope and sequence as well as learner readiness when presenting problem-solving activities.
- Be sure students have a firm understanding and error-free application of requisite skills to solve novel problems.
- Have instructional scaffolds in place that can be removed if or when students no longer need them.
- If you want to pique students’ interest at the beginning of the lesson by presenting a challenging and novel problem, allow limited time for exploration.
- Motivate students by effectively teaching them the skills to be successful.
(5) Understand Limitations of Growth Mindset:
- Focus mathematics instruction on building mathematics skills.
- Acknowledge that student engagement during instruction and willingness to put forth effort to engage in mathematics tasks is needed.
(6) Attend to Executive Functions:
- Teach explicitly.
- Segment skills and use clear language.
- Allow for high levels of opportunities to respond, which are connected to positive student outcomes.
(7) Use Timed Assessments Appropriately:
- Use assessments that target the student’s instructional range.
- Administer timed assessments as low-stakes activities.
- Embed goal setting as part of the process.
- If administering timed assessment class wide, ensure the floor is high enough for all students.
Endnotes
[1] Baum, S., Ma, J., & Payea, K. (2013). Education pays: The benefits of higher education for individuals and society. College Board.
[2] Davis-Kean, P., Domina, T., Kuhfeld, M., Ellis, A., & Gershoff, E. T. (2022). It matters how you start: Early numeracy mastery predicts high school math course-taking and college attendance. Infant and Child Development, 31(2), e2281. https://doi.org/10.1002/icd.2281
[3] Byun, S.-y., Irvin, M. J., & Bell, B. A. (2015). Advanced math course taking: Effects on math achievement and college enrollment. The Journal of Experimental Education, 83(4), 439-468. https://doi.org/10.1080/00220973.2014.919570
[4] You, S., & Nguyen, J. (2012). Multilevel analysis of student pathways to higher education. Educational Psychology, 32(7), 860-882. https://doi.org/10.1080/01443410.2012.746640
[5] Lee, M. (2012). College for all: Gaps between desirable and actual P-12 math achievement trajectories for college readiness. Educational Research, 41(2), 43-55. https://doi.org/10.3102/0013189X11432746
[6] Ritchie, S. J., & Bates, T. C. (2013). Enduring links from childhood mathematics and reading achievement to adult socioeconomic status. Psychological Science, 24(7), 1301-1308. https://doi.org/10.1177/0956797612466268
[7] Fyfe, E. R., Rittle-Johnson, B., & Farran, D. C. (2019). Predicting success on high-stakes math tests from preschool math measures among children from low-income homes. Journal of Educational Psychology, 111(3), 402-413.
[8] Nguyen, T., Watts, T. W., Duncan, G. J., Clements, D. H., Sarama, J. S., Wolfe, C., & Spitler, M. E. (2016). Which preschool mathematics competencies are most predictive of fifth grade achievement? Early Childhood Research Quarterly, 36, 550-560. https://doi.org/10.1016/j.ecresq.2016.02.003
[9] LeFevre, J.-A., Fast, L., Skwarchuk, S.-L., Smith-Chant, B. L., Bisanz, J., Kamawar, D., & Penner-Wilger, M. (2010). Pathways to mathematics: Longitudinal predictors of performance. Child Development, 81(6), 1753-1767. https://doi.org/10.1111/j.1467-8624.2010.01508.x
[10] Morgan, P. L., Farkas, G., & Wu, Q. (2011). Kindergarten children’s growth trajectories in reading and mathematics: Who falls increasingly behind? Journal of Learning Disabilities, 44(5), 472-488. https://doi.org/10.1177/0022219411414010
[11] Watts, T. W., Duncan, G. J., Siegler, R. S., & Davis-Kean, P. E. (2014). What’s past is prologue: Relations between early mathematics knowledge and high school achievement. Educational Research, 43(7), 352-360. https://doi.org/10.3102/0013189X14553660
[12] Jordan, N. C., Resnick, I., Rodrigues, J., Hansen, N., & Dyson, N. (2017). Delaware longitudinal study of fraction learning: Implications for helping children with mathematics difficulties. Journal of Learning Disabilities, 50(6), 621-630. https://doi.org/10.1177/0022219416662033
[13] Siegler, R. S., Duncan, G. J., Davis-Kean, P. E., Duckworth, K., Claessens, A., Engel, M. Susperreguy, M. I., & Chen, M. (2012). Early predictors of high school mathematics achievement. Psychological Science, 23(7), 691-697. https://doi.org/10.1177/0956797612440101
[14] Wei, X., Lenz, K. B., & Blackorby, J. (2013). Math growth trajectories of students with disabilities: Disability category, gender, racial, and socioeconomic status differences from ages 7 to 17. Remedial and Special Education, 34(3), 154-165. https://doi.org/10.1177%2F0741932512448253
[15] ibid
[16] Kohli, N., Sullivan, A. L., Sadeh, S., & Zopluoglu, C. (2015). Longitudinal mathematics development of students with learning disabilities and students without disabilities: A comparison of linear, quadratic, and piecewise linear mixed effects models. Journal of School Psychology, 53, 105-120. https://doi.org/10.1016/j.jsp.2014.12.002
[17] Clarke, B., Doabler, C. T., Smolkowski, K., Baker, S. K., Fien, H., & Cary, M. S. (2016). Examining the efficacy of a Tier 2 kindergarten mathematics intervention. Journal of Learning Disabilities, 49(2), 152–165. https://doi.org/10.1177/0022219414538514
[18] Fuchs, L. S., Powell, S. R., Cirino, P. T., Schumacher, R. F., Marrin, S., Hamlett, C. L., Fuchs, D., Compton, D. L., & Changas, P. C. (2014). Does calculation or word-problem instruction provide a stronger route to pre-algebraic knowledge? Journal of Educational Psychology, 106(4), 990–1006. https://doi.org/10.1037/a0036793
[19] Booth, J. L., McGinn, K. M., Barbieri, C., Begolli, K. N., Chang, D., Miller-Cotto, D., Young, L. K., & Davenport, J. L. (2016). Evidence for cognitive science principles that impact learning in mathematics. In D. C. Geary, D. B. Berch, R. Ochsendorf, & K. M. Koepke (Eds.), Acquisition of complex arithmetic skills and higher order mathematics concepts (pp. 297-325). Academic Press.
[20] Cook, B. G., & Cook, S. C. (2013). Unraveling evidence-based practices in special education. The Journal of Special Education, 47(2), 71-82. https://doi.org/10.1177/0022466911420877
[21] Rittle-Johnson, B., Schneider, M., & Star, J. R. (2015). Not a one-way street: Bidirectional relations between procedural and conceptual knowledge of mathematics. Educational Psychology Review, 27, 587-597. https://doi.org/10.1007/s10648-015-9302-x
[22] Stohlmann, M., Cramer, K., Moore, T., & Maiorca, C. (2014). Changing pre-service elementary teachers’ beliefs about mathematical knowledge. Mathematics Teacher Education and Development, 16(2), 4-24. https://eric.ed.gov/?id=EJ1052599
[23] National Council of Teachers of Mathematics. (2014). Principles to actions: Ensuring mathematical success for all.
[24] Witzel, B. S., Riccomini, P. J., & Schnieder, E. (2008). Implementing CRA with secondary students with learning disabilities in mathematics. Intervention in School and Clinic, 43(5), 270-278. https://doi.org/10.1177%2F1053451208314734
[25] Rittle-Johnson, B., Schneider, M., & Star, J. R. (2015). Not a one-way street: Bidirectional relations between procedural and conceptual knowledge of mathematics. Educational Psychology Review, 27, 587-597. https://doi.org/10.1007/s10648-015-9302-x
[26] Crooks, N. M., & Alibali, M. W. (2014). Defining and measuring conceptual knowledge in mathematics. Developmental Review, 34, 344-377. https://doi.org/10.1016/j.dr.2014.10.001
[27] Rittle-Johnson, B., & Alibali, M. W. (1999). Conceptual and procedural knowledge of mathematics: Does one lead to the other? The Journal of Educational Psychology, 91(1), 175-189. https://doi.org/10.1037/0022-0663.91.1.175
[28] Rittle-Johnson, B., Schneider, M., & Star, J. R. (2015). Not a one-way street: Bidirectional relations between procedural and conceptual knowledge of mathematics. Educational Psychology Review, 27, p. 595. https://doi.org/10.1007/s10648-015-9302-x
[29] Schneider, M., Rittle-Johnson, R., & Star, J. R. (2011). Relations among conceptual knowledge, procedural knowledge, and procedural flexibility in two samples differing in prior knowledge. Developmental Psychology, 47(6), 1525-1538. https://doi.org/10.1037/a0024997
[30] Fuchs, L. S., Powell, S. R., Cirino, P. T., Schumacher, R. F., Marrin, S., Hamlett, C. L., Fuchs, D., Compton, D. L., & Changas, P. C. (2014). Does calculation or word-problem instruction provide a stronger route to pre-algebraic knowledge? Journal of Educational Psychology, 106(4), 990–1006. https://doi.org/10.1037/a0036793
[31] Namkung, J. M., & Bricko, N. (2021). The effects of algebraic equation solving intervention for students with mathematics learning difficulties. Journal of Learning Disabilities, 54(2), 111-123. https://doi.org/10.1177/0022219420930814
[32] Star, J. R. (2005). Reconceptualizing procedural knowledge. Journal for Research in Mathematics Education, 36(5), 404-411. https://doi.org/10.2307/30034943
[33] Rittle-Johnson, B. (2017). Developing mathematics knowledge. Child Development Perspectives, 11(3), 184-190. https://doi.org/10.1111/cdep.12229
[34] Kamii, C., & Dominick, A. (1997). To teach or not to teach algorithms. Journal of Mathematical Behavior, 16(1), p. 60. https://doi.org/10.1016/S0732-3123(97)90007-9
[35] Fischer, J.-P., Vilette, B., Joffredo-Lebruin, S., Morellato, M., La Normand, C., Scheibling-Seve, C., & Richard, J. F. (2019). Should we continue to teach standard written algorithms for the arithmetical operations? The example of subtraction. Educational Studies in Mathematics, 101, 105-121. https://doi.org/10.1007/s10649-019-09884-9
[36] Kamii, C., & Dominick, A. (1997). To teach or not to teach algorithms. Journal of Mathematical Behavior, 16(1), 51-61. https://doi.org/10.1016/S0732-3123(97)90007-9
[37] National Council of Teachers of Mathematics. (2014). Principles to actions: Ensuring mathematical success for all.
[38] Fuson, K. C., & Beckmann, S. (2013). Standard algorithm in the Common Core state standards. National Council of School Mathematics Journal of Mathematics Education Leadership, 14(2), 14-30.
[39] Alfieri, L., Brooks, P. J., Aldrich, N. J., & Tenebaum, H. R. (2011). Does discovery-based instruction enhance learning? Journal of Educational Psychology, 103(1), 1-18. https://doi.org/10.1037/a0021017
[40] Torbeyns, J., & Verschaffel, L. (2016). Mental computation or standard algorithm? Children’s strategy choices on multi-digit subtractions. European Journal of Psychology of Education, 31(2), 99-116. https://doi.org/10.1007/s10212-015-0255-8
[41] Norton, S. (2012). The use of alternative algorithms in whole number computation. Interventional Journal for Mathematics Teaching and Learning, 2-16.
[42] Fischer, J.-P., Vilette, B., Joffredo-Lebruin, S., Morellato, M., La Normand, C., Scheibling-Seve, C., & Richard, J. F. (2019). Should we continue to teach standard written algorithms for the arithmetical operations? The example of subtraction. Educational Studies in Mathematics, 101, 105-121. https://doi.org/10.1007/s10649-019-09884-9
[43] Thanheiser, E. (2009). Preservice elementary school teachers’ conceptions of multidigit whole numbers. Journal for Research in Mathematics Education, 40(3), 251-281. https://doi.org/10.5951/jresematheduc.40.3.0251
[44] Rodríguez-Sánchez, M. M., Sánchez-García, A. B., & López-Fernáandez, R. (2020). Subtraction: More than an algorithm? Sustainability, 12, 9148. https://doi.org/10.3390/su12219148
[45] Nelson, G., & Powell, S. R. (2018). Computation error analysis: Students with mathematics difficulty compared to typically achieving students. Assessment for Effective Intervention, 43(3), 144-156. https://doi.org/10.1177/1534508417745627
[46] Fuchs, L. S., Powell, S. R., Hamlett, C. L., Fuchs, D., Cirino, P. T., & Fletcher, J. M. (2008). Remediating computational deficits at third grade: A randomized field trial. Journal of Research on Educational Effectiveness, 1(1), 2-32. https://doi.org/10.1080/19345740701692449
[47] Zhang, D., Xin, Y. P., Harris, K., & Ding, Y. (2014). Improving multiplication strategic development in children with math difficulties. Learning Disability Quarterly, 37(1), 15-30. https://doi.org/10.1177/0731948713500146
[48] Gersten, R., Ferrini-Mundy, J., Benbow, C., Clements, D., Loveless, T., Williams, V., Arispe, I., & Banfield, M. (2008). Report of the task group on instructional practices (National Mathematics Advisory Panel). http://www.ed.gov/about/bdscomm/list/mathpanel/report/instructional-practices.pdf
[49] Chong, S. L., & Siegel, L. S. (2008). Stability of computational deficits in math learning disability from second through fifth grades. Developmental Neuropsychology, 33(3), 300-317. https://doi.org/10.1080/87565640801982387
[50] Koponen, T., Aunola, K., Ahonen, T., & Nurmi, J.-E. (2007). Cognitive predictors of single-digit and procedural calculation skills and their covariation with reading skill. Journal of Experimental Child Psychology, 97(3), 220-241. https://doi.org/10.1016/j.jecp.2007.03.001
[51] Fuchs, L. S., Fuchs, D., Seethaler, P. M., & Barnes, M. A. (2020). Addressing the role of working memory in mathematical word-problem solving when designing intervention for struggling learners. ZDM Mathematics, 52, 87-96. https://doi.org/10.1007/s11858-019-01070-8
[52] Söderqvist, S., & Nutley, S. B. (2014). Working memory training is associated with long term attainments in math and reading. Frontiers in Psychology, 6, 1711. https://doi.org/10.3389/fpsyg.2015.01711
[53] Tan, P., Padilla, A., & Lambert, R. (2022). A critical review of educator and disability research in mathematics education: A decade of dehumanizing waves and humanizing wakes. Review of Education Research. https://doi.org/10.3102/00346543221081874
[54] Kirschner, P. A., Sweller, J., & Clark, R. E. (2006). Why minimal guidance during instruction does not work: An analysis of the failure of constructivist, discovery, problem-based, experiential, and inquiry-based teaching, Educational Psychologist, 41(2), 75-86. https://doi.org/10.1207/s15326985ep4102_1
[55] Alfieri, L., Brooks, P. J., Aldrich, N. J., & Tenebaum, H. R. (2011). Does discovery-based instruction enhance learning? Journal of Educational Psychology, 103(1), 1-18. https://doi.org/10.1037/a0021017
[56] Carbonneau, K. J., Marley, S. C., & Selig, J. P. (2013). A meta-analysis of the efficacy of teaching mathematics with concrete manipulatives. Journal of Educational Psychology, 105(2), 380–400. https://doi.org/10.1037/a0031084
[57] De Jong, T., & Van Joolingen, W. R. (1998). Scientific discovery learning with computer simulations of conceptual domains. Review of Educational Research, 68, 179–202. https://doi.org/10.3102%2F00346543068002179
[58] Hermann, G. (1969). Learning by discovery: A critical review of studies. Journal of Experimental Education, 38, 58–72. https://doi.org/10.1080/00220973.1969.11011167
[59] Lazonder, A. W., & Harmsen, R. (2016). Meta-analysis of inquiry-based learning: Effects of guidance. Review of Educational Review, 86(3), 681-718.
[60] De Jong, T., & Van Joolingen, W. R. (1998). Scientific discovery learning with computer simulations of conceptual domains. Review of Educational Research, 68, 179–202. https://doi.org/10.3102%2F00346543068002179
[61] Archer, A., & Hughes, C. (2011). Explicit instruction: Effective and efficient teaching. Guilford.
[62] Lazonder, A. W., & Harmsen, R. (2016). Meta-analysis of inquiry-based learning: Effects of guidance. Review of Educational Review, 86(3), 681-718.
[63] Kalyuga, S. (2007). Expertise reversal effect and its implications for learner-tailored instruction. Educational Psychology Review, 19, 509-539.
[64] Hughes, C. A., Morris, J. R., Therrien, W. J., & Benson, S. K. (2017). Explicit instruction: Historical and contemporary contexts. Learning Disabilities Research and Practice, 32(3), 140-148. https://doi.org/10.1111/ldrp.12142
[65] Alfieri, L., Brooks, P. J., Aldrich, N. J., & Tenebaum, H. R. (2011). Does discovery-based instruction enhance learning? Journal of Educational Psychology, 103(1), 1-18. https://doi.org/10.1037/a0021017
[66] De Jong, T., & Van Joolingen, W. R. (1998). Scientific discovery learning with computer simulations of conceptual domains. Review of Educational Research, 68, 179–202. https://doi.org/10.3102%2F00346543068002179
[67] Hermann, G. (1969). Learning by discovery: A critical review of studies. Journal of Experimental Education, 38, 58–72. https://doi.org/10.1080/00220973.1969.11011167
[68] Lazonder, A. W., & Harmsen, R. (2016). Meta-analysis of inquiry-based learning: Effects of guidance. Review of Educational Review, 86(3), 681-718.
[69] Nelson, G., Cook, S. C., Zarate, K., Drake, K. R., Powell, S. R., Maggin, D. M., Drake, K. R., Kiss, A. J., Ford, J. W., Sun, L., & Espinas, D. R. (in press). A systematic review of meta-analyses in special education: Exploring the evidence base for high-leverage practices. Remedial and Special Education. https://doi.org/10.1177/07419325211063491
[70] Doabler, C. D., & Fien, H. (2014). Explicit mathematics instruction: What teachers can do for teaching students with mathematics difficulties. Intervention in School and Clinic, 48(5), 276-285. https://doi.org/10.1177/1053451212473151
[71] Hughes, C. A., Morris, J. R., Therrien, W. J., & Benson, S. K. (2017). Explicit instruction: Historical and contemporary contexts. Learning Disabilities Research and Practice, 32(3), 140-148. https://doi.org/10.1111/ldrp.12142
[72] Archer, A., & Hughes, C. (2011). Explicit instruction: Effective and efficient teaching. Guilford.
[73] Haydon, T. G., Mancil, R., Kroeger, S. D., McLeskey, J., & Lin, W-Y. (2011). A review of the effectiveness of guided notes for students who struggle learning academic content. Preventing School Failure: Alternative Education for Children and Youth, 55(4), 226-231. https://doi.org/10.1080/1045988X.2010.548415
[74] Popham, M., Adams, S., & Hodge, J. (2020). Self-regulated strategy development to teach mathematics problem solving. Intervention in School and Clinic, 55(3) 154-161. https://doi.org/10.1177%2F1053451219842197
[75] Carbonneau, K. J., Marley, S. C., & Selig, J. P. (2013). A meta-analysis of the efficacy of teaching mathematics with concrete manipulatives. Journal of Educational Psychology, 105(2), 380–400. https://doi.org/10.1037/a0031084
[76] Peltier, C. Morin, K. L., Bouck, E. C., Lingo, M. E., Pulos, J. M., Scheffler, F. A., Suk, A. Mathews, L. A., Sinclair, T. E., & Deardorff, M. E. (2020). A meta-analysis of single-case research using mathematics manipulatives with students at risk or identified with a disability. The Journal of Special Education, 54(1) 3-15. https://doi.org/10.1177/0022466919844516
[77] Lemley, S. M., Ivy, J. T., Franz, D. P., & Oppenheimer, S. F. (2019) Metacognition and middle grade mathematics teachers: Supporting productive struggle. The Clearing House: A Journal of Educational Strategies, Issues and Ideas, 92(1-2), 15-22, DOI: 10.1080/00098655.2018.1547264
[78] Kapur, M. (2011). A further study of productive failure in mathematical problem solving: unpacking the design components. Instructional Science, 39(4), 561–579.
[79] Hiebert, J., & Grouws, D. A. (2007). The effects of classroom mathematics teaching on students’ learning. In F. K. Lester (Ed.), Second handbook of research on mathematics teaching and learning (p 387). Information Age.
[80] Hiebert, J., & Grouws, D. A. (2007). The effects of classroom mathematics teaching on students’ learning. In F. K. Lester (Ed.), Second handbook of research on mathematics teaching and learning (p 387). Information Age.
[81] National Council of Teachers of Mathematics. (2014). Principles to actions: Ensuring mathematical success for all.
[82] Kirschner, P. A., Sweller, J., & Clark, R. E. (2006). Why minimal guidance during instruction does not work: An analysis of the failure of constructivist, discovery, problem-based, experiential, and inquiry-based teaching, Educational Psychologist, 41(2), 75-86. https://doi.org/10.1207/s15326985ep4102_1
[83] Losinski, M., Ennis, R. P., Sanders, S., & Wiseman, N. (2019). An investigation of SRSD to teach fractions to students with disabilities. Exceptional Children, 85(3), 291-308. https://doi.org/10.1177/0014402918813980
[84] Popham, M., Adams, S., & Hodge, J. (2020). Self-regulated strategy development to teach mathematics problem solving. Intervention in School and Clinic, 55(3) 154-161. https://doi.org/10.1177%2F1053451219842197
[85] Hughes, E. M., & Lee, J. Y. (2020). Effects of a mathematical writing intervention on middle school students’ performance. Reading & Writing Quarterly, 36(2), 176-192, https://doi.org/10.1080/10573569.2019.1677537
[86] Kapur, M. (2014). Productive failure in learning math. Cognitive Science A Multidisciplinary Journal, 38(5), 1008-1022. https://doi-org.ezaccess.libraries.psu.edu/10.1111/cogs.12107
[87] Schneider, M., Rittle-Johnson, R., & Star, J. R. (2011). Relations among conceptual knowledge, procedural knowledge, and procedural flexibility in two samples differing in prior knowledge. Developmental Psychology, 47(6), 1525-1538. https://doi.org/10.1037/a0024997
[88] Hiebert, J., & Carpenter, T. P. (1992). Learning and teaching with understanding. In D. A. Grouws (Ed.), Handbook of research on mathematics teaching and learning: A project of the National Council of Teachers of Mathematics (pp. 65-97). Macmillan.
[89] Ashman, G., Kalyuga, S., & Sweller, J. (2020). Problem-solving or explicit instruction: Which should go first when element interactivity is high?. Educational psychology review, 32(1), 229-247. https://doi.org/10.1007/s10648-019-09500-5
[90] Hughes, E. M., & Riccomini, P. J. (2011). Mathematics motivation and self-efficacy of middle school students. Focus on Middle School, 24(1), 1-6.
[91] Kirschner, P. A., Sweller, J., & Clark, R. E. (2006). Why minimal guidance during instruction does not work: An analysis of the failure of constructivist, discovery, problem-based, experiential, and inquiry-based teaching, Educational Psychologist, 41(2), 75-86. https://doi.org/10.1207/s15326985ep4102_1
[92] Schwonke, R., Renkl, A., Krieg, C., Wittwer, J., Aleven, V., & Salden, R. (2009).The worked-example effect: Not an artefact of lousy control conditions. Computers in Human Behavior, 25(2), 258-266. https://doi.org/10.1016/j.chb.2008.12.011.
[93] Weiner, B., & Kukla, A. (1970). An attributional analysis of achievement motivation. Journal of personality and Social Psychology, 15(1), 1-20. https://psycnet.apa.org/doi/10.1037/h0029211
[94] Elliott, E. S., & Dweck, C. S. (1988). Goals: An approach to motivation and achievement. Journal of Personality and Social Psychology, 54(1), 5-12. https://psycnet.apa.org/doi/10.1037/0022-3514.54.1.5
[95] Dweck, C. S. (1999). Self-Theories: Their role in motivation, personality and development. Taylor and Francis/Psychology Press.
[96] Yeager, D. S., Hanselman, P., Walton, G. M., Murray, J. S., Crosnoe, R., Muller, C., & Dweck, C. S. (2019). A national experiment reveals where a growth mindset improves achievement. Nature, 573(7774), 364-369. https://doi.org/10.1038/s41586-019-1466-y
[97] Li, Y., & Bates, T. C. (2020). Testing the association of growth mindset and grades across a challenging transition: Is growth mindset associated with grades?. Intelligence, 81, 101471. https://doi.org/10.1016/j.intell.2020.101471
[98] Fuchs, L. S., Wang, A. Y., Preacher, K. J., Malone, A. S., Fuchs, D., & Pachmayr, R. (2021). Addressing challenging mathematics standards with at-risk learners: A randomized controlled trial on the effects of fractions intervention at third grade. Exceptional Children, 87(2), 163-182. https://doi.org/10.1177%2F0014402920924846
[99] Diamond, A. (2006). The early development of executive functions. In E. Bialystok & F. I. M. Craik (Eds.), Lifespan cognition: Mechanisms of change (pp. 70–95). Oxford University Press. https://doi.org/10.1093/acprof:oso/9780195169539.003.0006
[100] Jacob, R., & Parkinson, J. (2015). The potential for school-based interventions that target executive function to improve academic achievement: A review. Review of Educational Research, 85(4), 512-552. https://doi.org/10.3102%2F0034654314561338
[101] Jacob, R., & Parkinson, J. (2015). The potential for school-based interventions that target executive function to improve academic achievement: A review. Review of Educational Research, 85(4), 512-552. https://doi.org/10.3102%2F0034654314561338
[102] Kassai, R., Futo, J., Demetrovics, Z., & Takacs, Z. K. (2019). A meta-analysis of the experimental evidence on the near-and far-transfer effects among children’s executive function skills. Psychological Bulletin, 145(2), 165-188. https://psycnet.apa.org/doi/10.1037/bul0000180
[103] Abreu-Mendoza, R. A., Chamorro, Y., Garcia-Barrera, M. A., & Matute, E. (2018). The contributions of executive functions to mathematical learning difficulties and mathematical talent during adolescence. PLoS ONE, 13(12), e0209267. https://doi.org/10.1371/journal.pone.0209267
[104] Jacob, R., & Parkinson, J. (2015). The potential for school-based interventions that target executive function to improve academic achievement: A review. Review of Educational Research, 85(4), 512-552. https://doi.org/10.3102%2F0034654314561338
[105] Hughes, C. A., Morris, J. R., Therrien, W. J., & Benson, S. K. (2017). Explicit instruction: Historical and contemporary contexts. Learning Disabilities Research and Practice, 32(3), 140-148. https://doi.org/10.1111/ldrp.12142
[106] Smith, J. L. M., Sáez, L., & Doabler, C. T. (2016). Using explicit and systematic instruction to support working memory. TEACHING Exceptional Children, 48(6), 275-281. https://doi.org/10.1177%2F0040059916650633
[107] Hughes, E. M., Powell, S. R., & Stevens, E. A. (2016). Supporting clear and concise mathematics language: Say this, not that. Teaching Exceptional Children, 49(1), 1-7. https://doi.org/10.1177/0040059916654901
[108] Powell, S., Hughes, E. M., & Stevens, E. (2019). Math language in middle school: Be more specific. Teaching Exceptional Children, 51(4), 286-295. https://doi.org/10.1177/0040059918808762
[109] MacSuga-Gage, A. S., & Simonsen, B. (2015). Examining the effects of teacher-directed opportunities to respond on student outcomes: A systematic review of the literature. Education and Treatment of Children, 38(2), 211–239. http://www.jstor.org/stable/44683954
[110] Burns, M. K., VanDerHeyden, A. M., & Jiban, C. L. (2006). Assessing the instructional level for mathematics: A comparison of methods. School Psychology Review, 35(3), 401-418. https://doi.org/10.1080/02796015.2006.12087975
[111] Binder, C. (1996). Behavioral fluency: Evolution of a new paradigm. The Behavior Analyst, 19(2), 163-197. https://doi.org/10.1007/BF03393163
[112] Schumacher, R. F., Zumeta Edmonds, R., & Arden, S. V. (2017). Examining implementation of intensive intervention in mathematics. Learning Disabilities Research and Practice, 32(3), 189-199. https://doi.org/10.1111/ldrp.12141
[113] Sweller, J., & Chandler, P. (1991). Evidence for cognitive load theory. Cognition and Instruction, 8(4), 351-362. https://doi.org/10.1207/s1532690xci0804_5
[114] Burns, M. K., Codding, R. S., Boice, C. H., & Lukito, G. (2010). Meta-analysis of acquisition and fluency math interventions with instructional and frustration level skills: Evidence for a skill-by-treatment interaction. School Psychology Review, 39(1), 69-83. https://doi.org/10.1080/02796015.2010.12087791
[115] Willingham, D. T., Hughes, E. M., & Dobolyi, D. G. (2015). The scientific status of learning styles theories. Teaching of Psychology, 42(3), 266–271.
ALSO READ by David Geary: Setting the preschool foundation for success in Mathematics
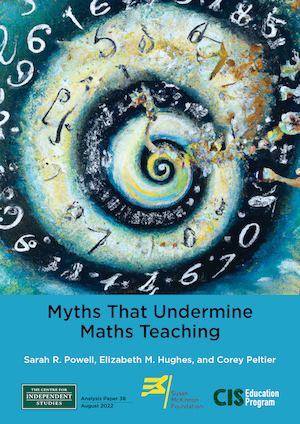